
Artikel
02
mei
From Data to Discovery: AI’s Revolutionary Impact on Upstream Oil and Gas Transformation
Artificial Intelligence (AI), recognized as a pivotal technology, is rapidly transforming various industries, offering substantial potential for innovation and growth. Beyond digital and tech savvy sectors, latecomers like oil and gas and oilfield service providers are increasingly relying on AI solutions. Although initial considerations for AI in oil and gas trace back to the 1970s, the industry has recently become more proactive in seeking AI applications. As per an Ernst & Young survey, 92% of global oil and gas companies are currently investing in or have plans to invest in AI within the next two years.
The oil and gas sector is categorized into “upstream, midstream, and downstream”. This article focuses on the upstream sector, which is the most capital-intensive and vital segment within the oil and gas industry. Upstream activities deal with the subsurface aspects, encompassing exploration, field development, and the production of crude oil and gas.
Traditional methods often struggle to handle the intricacies of the upstream sector. AI can enhance predictive modeling, optimize drilling operations, and provide valuable insights into reservoir behavior, thereby offering a transformative approach to the challenges faced by the oil and gas upstream industry. Some major use cases of AI in improving oil and gas upstream activities are as follows:
Forecasting Oil Production Using Advanced Machine Learning
Machine learning (ML) is a branch of AI. Its core attention is on the formulation of algorithms and statistical models that enable computer systems to learn from data and enhance their performance over time. In other words, it empowers systems to automatically adapt and make decisions based on experience without explicit programming. The relationship between machine learning and AI lies in the fact that machine learning techniques contribute significantly to the development of intelligent systems, allowing them to analyze patterns, make predictions, and solve complex problems.
Oil and gas companies spend a lot of money and expertise to make sure they get the most out of their gas wells. It involves looking at a ton of information, but it’s often a trade-off between how fast they can analyze it and how accurate the analysis is. Machine Learning can make this process faster and more effective because it automatically learns from the data, including daily gas production, well logs, fluid information, and more, without any specific instructions. It also helps experts in finding out the best settings for the gas wells to produce the most gas, helping the company save time and make their operations more efficient.
Secondly, experts often use traditional models to guess how much oil and gas will be produced. Despite fitting well with historical data, these models yield varied forecasts, introducing a level of inconsistency or uncertainty in the predictions they generate. In contrast, ML emerges as a promising advancement in the prediction of oil and gas production. It effectively manages uncertainties in production estimates, providing predictions that are more accurate and adaptable.
Prediction of Stuck Pipe Incidents
A significant challenge in drilling operations is the occurrence of a stuck pipe problem, where the drill pipe can’t move freely in the well bore. In other words, a stuck pipe is when the drill is stuck and can’t move. It slows everything down and costs a lot of money. Traditional methods for analyzing rapid and high-dimensional data for predicting stuck pipe incidents are inefficient. With the help of ML, experts can predict and address the stuck pipe challenge efficiently in the upstream sector of the oil and gas industry. It can do this by identifying significant patterns within the data and making predictions based on the provided information.
Pre-Drilling Risk Prediction
Oil and gas play a vital role in advancing human civilization. Drilling for these resources is a complicated task. It requires in-depth knowledge of well bore behavior for successful completion. AI can play a crucial role in the planning phase of drilling operations by analyzing a wealth of historical data, including past drilling experiences and the geological attributes of the drilling site. Anticipating and preventing potential issues through proactive problem avoidance helps experts recognize and mitigate challenges, such as encountering exceptionally hard ground, before they become significant during the drilling process.
Moreover, it can provide early warnings that enable companies to devise more informed and efficient drilling strategies. This approach results in cost savings and minimizes unexpected interruptions, facilitating smoother operations that adhere to timelines effectively. Addressing potential issues in advance not only enhances safety in the work environment but also allows companies to optimize the allocation of resources, encompassing both manpower and equipment. By doing so, resources are utilized more efficiently.
Synthetic Data Generation Using Generative AI
Generative AI is a subset of AI. It develops systems that can create new content, including images and text. Unlike traditional AI systems that are trained to identify trends or decision making using existing data, generative AI can generate novel outputs by learning from the patterns and structures present in the data it was trained on.
Accurate prediction of oil and gas production necessitates the availability of a complete dataset. Managing missing data is essential for ensuring the accuracy and dependability of predictions. Here, generative AI emerges as a solution by creating synthetic data that closely mimics genuine datasets. It does this by generating instances of absent data within complete datasets and evaluating the authenticity of the generated values through comparison with the original dataset.
By effectively mitigating the impact of missing data, Generative AI contributes to more accurate and reliable oil and gas production forecasting. Rodrigo and his team used this method to generate synthetic seismic images from sketches to provide oil and gas companies with top quality annotated data for predictions in their upstream activities. As a result, they successfully obtained a large dataset for precise predictions.
Conversational Chatbot for Drilling and Production Analytics
Energy companies require intelligent insights from their extensive datasets to enhance their operational efficiency. A Generative AI-enabled conversational chatbot designed for drilling and production analytics holds the promise of substantial improvement in the oil and gas upstream sector. It can enhance understanding of operations and provide instant access to insights by handling data efficiently. Its diagnostic analysis capabilities allow proactive issue identification, while the generation of operational recommendations contributes to efficiency.
The chatbot’s real-time responses can reduce the time and resources needed for manual tasks. Moreover, its continuous learning ensures ongoing refinement in providing relevant and valuable information. Ajay Singh, along with his colleagues, developed the smart chatbot capable of handling historical PDF drilling reports, a task typically challenging for information extraction and large-scale analysis. The chatbot played a key role in discovering that a particular method of extracting oil from wells was more efficient. As a result of this discovery, there was a notable improvement, with a substantial 20% increase in oil production.
Predictive Maintenance
By transforming historical data into actionable insights, Generative AI can empower oil and gas companies to enhance the reliability and efficiency of their equipment maintenance practices. It leverages historical maintenance and sensor data associated with infrastructure equipment such as pumps, compressors, and turbines. By analyzing this data, it creates predictive maintenance models. These models possess the capability to anticipate potential failures in the equipment before they happen. The proactive nature of these predictions provides early warnings that enable companies to minimize downtime, reduce maintenance expenditures, and optimize the distribution of technician resources.
Conclusion
While AI presents a variety of opportunities for revolutionizing oil and gas upstream activities, it is not without its limitations. One significant challenge lies in the dependence on the quality and quantity of available data for training. AI models require extensive and representative datasets to produce accurate and reliable predictions. In the oil and gas sector, obtaining comprehensive and diverse datasets can be challenging due to the proprietary nature of some data, potential data gaps, and the dynamic nature of subsurface conditions.
Furthermore, the interpretability of AI models remains a concern, as the complex algorithms involved may not provide transparent insights into the decision-making process. Ensuring the ethical use of AI in sensitive industries like oil and gas is another crucial consideration. As these technologies continue to evolve, addressing these limitations will be essential to maximize their effectiveness and ethical implementation in upstream oil and gas operations.
Disclaimer: The author is completely responsible for the content of this article. The opinions expressed are their own and do not represent IEEE’s position nor that of the Computer Society nor its Leadership.
What's your reaction ?
Follow us on Social Media
Recent posts
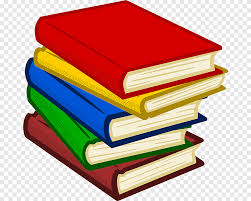
November 20, 2024
Books Article
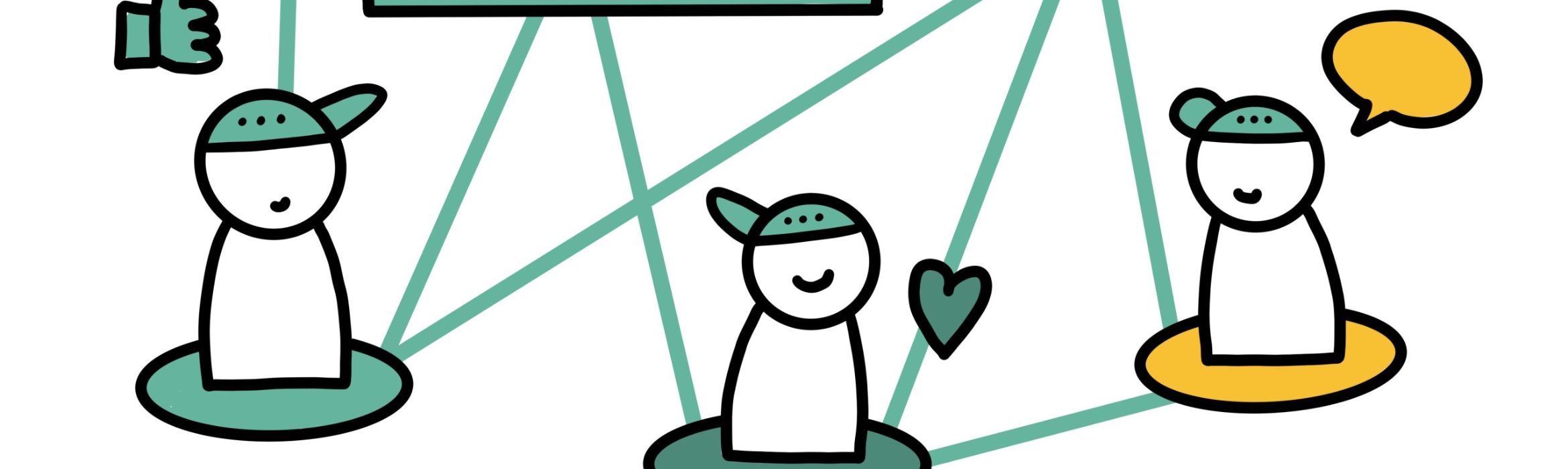
November 19, 2024
Hoe Aristoteles nu zorgt voor impactvolle communicatie
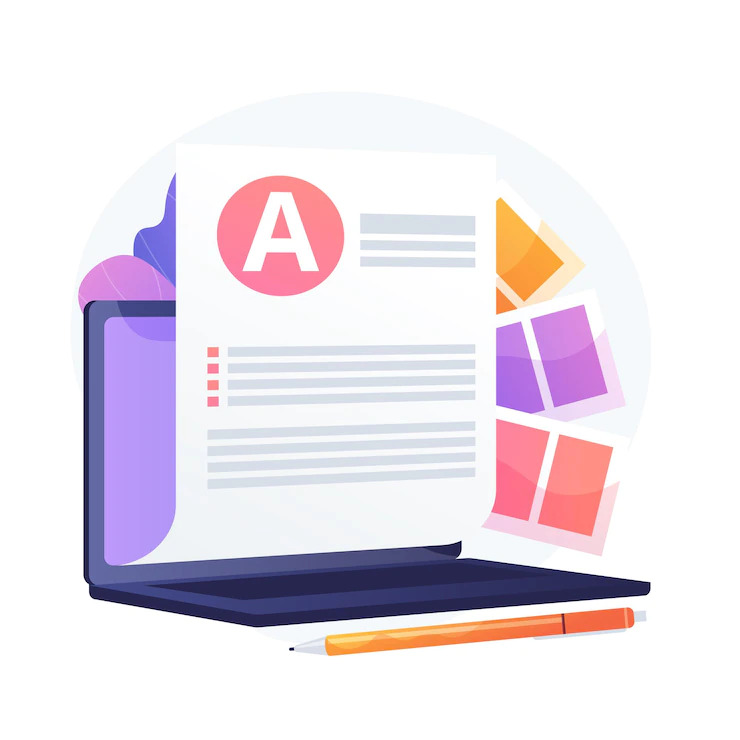
November 16, 2024
Loonkosten 2025: hier kun je als werkgever op rekenen
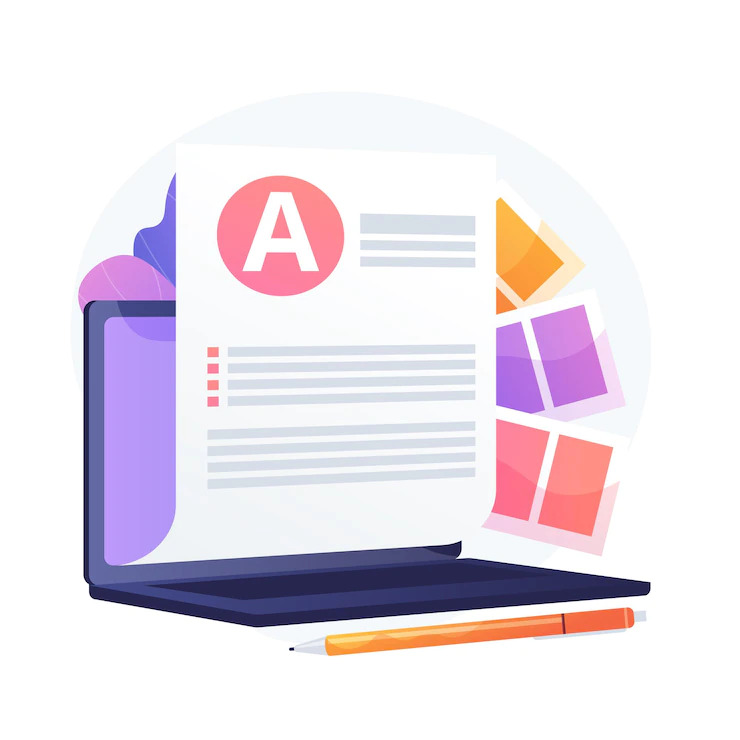
November 09, 2024
Dit betekent het regeerakkoord voor jou als ondernemer
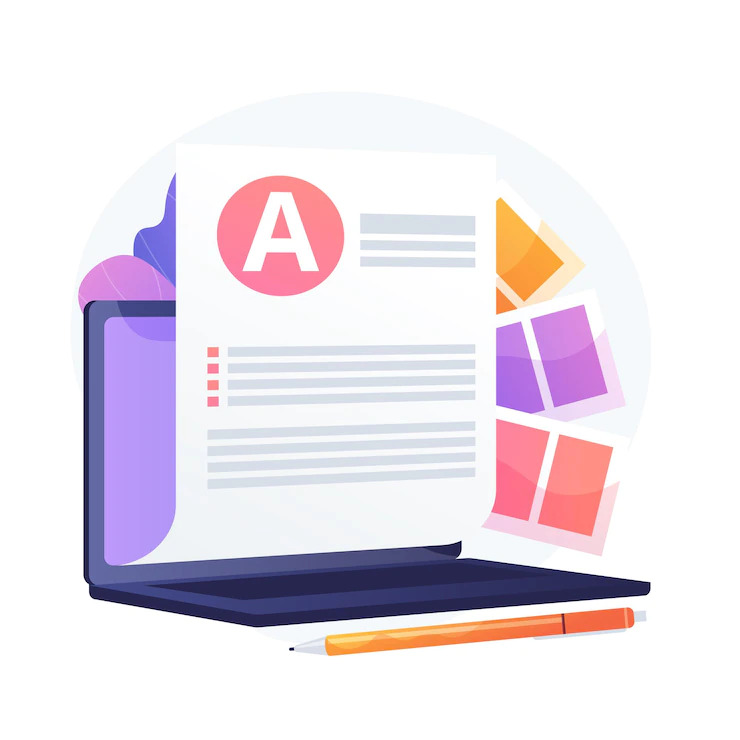
November 03, 2024
Dit betekent het regeerakkoord voor jou als ondernemer
Comments (0)
No reviews found
Add Comment